In this work, we set up a novel task of playlist context prediction. From a large playlist title corpus, we manually curate a subset of multilingual labels referring to user activities (e.g. “jogging”, “meditation”, “au calme”), which we further consider in the prediction task.
We explore different approaches to calculate and aggregate track-level contextual semantic embeddings in order to represent a playlist and predict the playlist context from this representation. Our baseline results show that the task can be addressed with a simple framework using information from either audio or distributional similarity of tracks in terms of track-context co-occurrences.
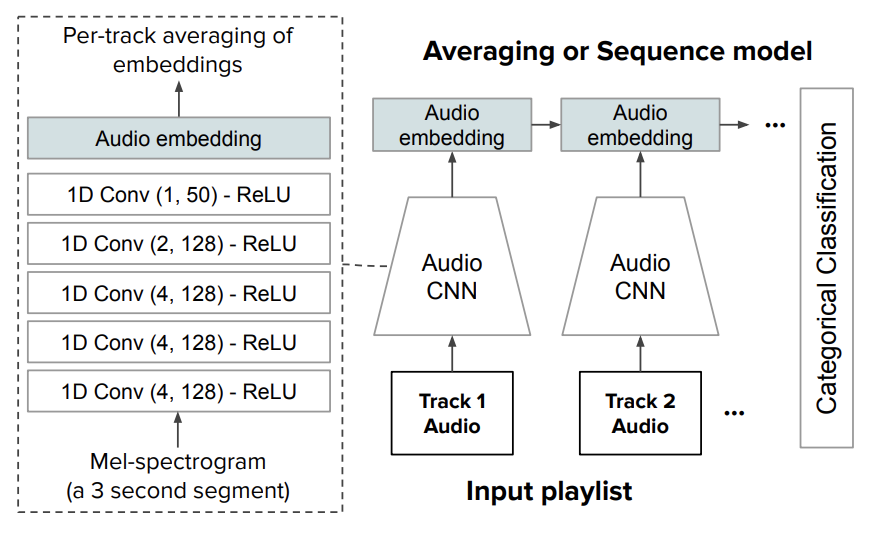
This work has been presented at the First Workshop on NLP for Music and Audio (NLP4MusA), co-located with ISMIR 2020. It is an extended version of Jeong Choi’s research internship at Deezer in 2019.